Why don’t polygenic scores work well in all groups?
A new study finds that it's not due to family-related confounding.
Written by Emil O. W. Kirkegaard.
Practically all human variation has a genetic component. In the fields of quantitative genetics and behavior genetics, scientists aim to quantify how much variation is caused by genes, as opposed to environmental factors (such as the family environment) and random noise. Heritability varies from relatively low (e.g., homosexuality) to very large (e.g., height), with most traits showing some sizeable but intermediate amount.
In my view, the main achievement of quantitative genetics and genomics over the last 10 years has been the development of polygenic scores (also known as polygenic indices or genetic risk scores). These are predictions of people’s genetic potential for some trait based on the results of GWAS or genome-wide association studies.
Such studies search across the entire genome and identify variants that are associated with variation in the trait in question. The way this is done is that each locus or position along the genome is tested for an association with the trait, usually when controlling for variables such as age, sex and genetic “principal components”. The latter serve as controls for any kind of unmeasured differences in ancestry or population structure that might exist in the dataset. Armed with the complete set of genome-wide associations, typically between 1–10 million, a model weights them in such a way as to best explain variation in the trait. The outcome is a polygenic score.
This process is similar to linear regression, but because of the sheer size of the dataset, a normal regression model cannot be used (there are more variables than cases in the dataset). Note that “polygenic” in this context simply denotes the fact that the relevant traits aren’t caused by single gene variants (as is the case for many rare disorders), but rather by hundreds or thousands of variants spread across the genome.
After the polygenic score has been spat out by the model, a new dataset is found for testing purposes. The polygenic score is calculated for each subject in the dataset and then compared with their known trait values. The association between known and predicted trait values quantifies its accuracy, typically in the form of a correlation or squared correlation.
Almost all GWAS have been done on samples of Europeans (that is, people of overwhelmingly European ancestry, regardless of where they live). However, it was soon discovered that when the models trained on these samples were applied to non-European samples, the accuracy was much lower. Why?
There are several different possible reasons. Maybe the genetic architecture of diverse human populations differs so much that variants which cause, say, taller height in Europeans do not cause taller height in other populations (or even cause shorter height). If so, this would mean that populations are even more genetically different than previously thought – that their genomes work in fundamentally different ways. However, this seems unlikely to be true.
The human genome has about 3 billion base pairs. Most of these don’t vary between humans. For example, we all have 2 C’s at position chr3:122,414,117. The variation that does exist may or may not affect the traits we care about; only large studies can tell us. The most widely used technology for measuring genetic variation in humans is the “array”. Despite being cheap and reliable, it can only measure certain kinds of variation and only at certain positions. Due to cost constraints, almost all data used for training models comes from arrays.
This turns out to be very important because many genetic signals (i.e., associations of specific positions along the genome with the trait of interest) are not causal at all. Rather, these positions are in close proximity to some other position, and it is there that the true causal variant lies. The position we happened to have measured is merely a stand-in, or proxy.
Positions along the genome that are statistically correlated are said to be in linkage disequilibrium (LD). Since the model cannot figure out from the data whether a position it has identified is causal or not, the proxies end up getting included in the polygenic score. They are said to “tag” the causal variant, and for this reason also called “tag variants” (they “tag along” with the other variant during the process of recombination due to their close proximity).
Consider the example of using dark, straight hair to predict a preference for noodle soup. There’s no real causal connection between the color and texture of someone’s hair to their food preferences. However, Asians happen to like noodles, and they also happen to have that dark, straight hair. And here’s the crux: the correlations, or LD, between different positions along the genome vary between populations (the reasons why are beyond the scope of this article).
It may be that in Europeans a given variant serves as an excellent proxy for some unknown causal variant, while in non-Europeans it does no such thing. Since the model is trained on Europeans, it will identify the proxies that work best in Europeans, regardless of whether they work well in the other populations. And the more distant those other populations are from Europeans, the less overlap there will be. This is called LD decay. The correlations between tag variants and causal variants “decay” with increasing genetic distance from the population in which the tag variants were identified.
But this still doesn’t exhaust the possible reasons why today’s polygenic scores are less accurate for non-Europeans.
Another is that genetic signals may actually be proxies for some environmental causal factors. It may be that a given variant happens to correlate with, say, living in London, and for some reason that affects the trait in question (dental damage from excessive tea drinking perhaps). Meanwhile in non-Europeans, the variant does not correlate with living in London, and therefore doesn’t predict anything. It could even be that some genetic variant happens to correlate with growing up in an intact family, and this is what explains its association with, say, clinical depression later in life. This would constitute an example of environmental confounding.
So how can scientists find out which reason is the correct one?
One way of doing so is to train the model only on data from siblings. Since they grew up in the same household, any genetic differences between them cannot be confounded by the family environment. The main issue with this approach is that few datasets consist of sibling pairs. Ironically, most datasets were initially designed to consist of unrelated persons – in order to maximize the amount of new information (measuring the genetics of both identical twins is generally not done because this would almost give us no additional information at all).
But with the decrease in cost of genotyping over the last couple of decades, we now have data on hundreds of thousands of full siblings. A new study used these data to examine whether models trained on sibling data are more accurate for non-Europeans. If they are, this would suggest that family-related confounding is a major reason why polygenic scores don’t generalise to other populations.
However, the researchers found that the models aren’t more accurate. Polygenic scores trained on European sibling data work just as poorly on Africans and admixed Americans (Hispanics) as those trained on data from unrelated Europeans:
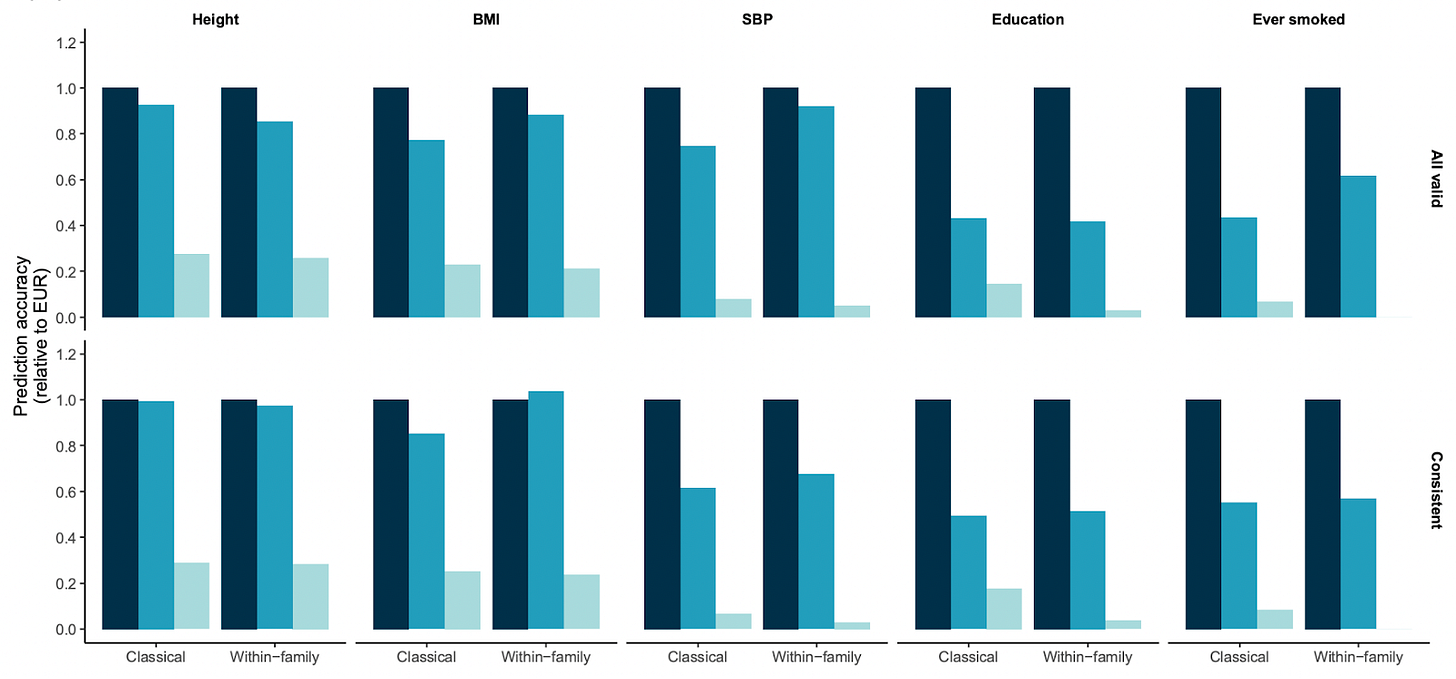
This finding suggests that family-related confounding is not a major reason why polygenic scores don’t generalise to other populations. The researchers did some further analyses to estimate how much of the difference in accuracy could be explained by LD decay.
For height and BMI, they find that about 90% of the difference could be explained by LD decay and differences in the frequencies of the genetic variants. But for three other traits, including educational attainment, it was only about 50%. The researchers compared people with mixed ancestry, and checked whether accuracy improved as a function of the percentage European ancestry. They found that it did, which makes it difficult to attribute the lack of generalizability to anything but genetics.
According to the researchers, the way forward is clear: we must collect more genetic data from families, especially siblings, in order to enhance our prediction capabilities further. We must also collect more data from non-Europeans, so that models can be trained that produce useful predictions for those populations too. The latter goal even has a “social justice” motivation because much of the research aims to construct clinically relevant predictions for, e.g., finding people at high risk for type 2 diabetes. (Since predictions currently work better for Europeans, any resulting interventions will be much more effective too – a genetic bias in healthcare that many are keen to eliminate).
Finally, the issue with tag variants makes it necessary to collect whole-genome sequencing data. Unlike arrays, these cover every position in the genome and therefore measure all the variants, including those missed by current datasets. Analysing such data should enable us to find the actual causal variants, which are crucial because they can be used for genetic editing. In 2023, the FDA approved two gene-editing drugs to cure people with severe genetic disorders like sickle-cell anemia. (There is undoubtedly more to come on this front.) Whole-genome sequencing data will also provide a definitive answer to the question about race differences in intelligence.
The new study rules out some implausible forms of environmental confounding and shows that LD decay is the main issue when comparing polygenic scores across groups. As usual, hereditarians were early to point this out (I wrote about it back in 2015).
Emil O. W. Kirkegaard is a social geneticist. You can follow his work on Twitter/X and Substack.
Consider supporting Aporia with a paid subscription:
You can also follow us on Twitter.
I appreciate your explanatory interpolations. Although I am ignorant of this branch (and most others) of science, it is enjoyable to read your accessible research results! Nice to learn something new. Thanks. Keep 'em coming.
Can't there just be different iq genes in different places. If you only measure iq genes from Europeans you're missing many other genes that contribute. So the more distantly related the more unique genes they have which you don't know what they do. You'd be missing half the picture.